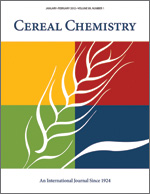
Cereal Chem 72:243-247 |
VIEW ARTICLE
Differentiation of Hard Red Wheat by Near-Infrared Analysis of Bulk Samples.
S. R. Delwiche, Y.-R. Chen, and W. R. Hruschka. Copyright 1995 by the American Association of Cereal Chemists, Inc.
Near-infrared reflectance spectroscopy (1,100-2,498 nm) has been used to identify hard red winter and hard red spring wheat classes. As a follow-up to a previous study which involved ground wheat samples, the authors have used the same samples on a whole kernel in-bulk (80 g) basis. Four years of U.S. winter and spring wheats were used. A small number (n = 150 samples per class) from the first three years' samples were used for calibration; the remaining portion (n = 1,325), plus all of the fourth year's samples (n = 778), were used to verify the models. Four types of classification algorithms were examined: multiple linear regression (MLR), principal component analysis with Mahalanobis distance (PCA/MD), partial least squares (PLS) analysis, and artificial neural networks (ANN). All four models demonstrated classification accuracies (defined as the percentage of correctly classified samples) greater than 88%, and most often, about 95% for samples grown during the same years as used in calibration. These accuracies were significantly better than those associated with discriminant models that were based solely on protein content, NIR-hardness, or a combination of protein and hardness. Spectrally sensed water-matrix interactions were probably beneficial to model accuracy; however, moisture content alone was not deemed necessary to a model's success. When predicting the fourth year, the MLR model needed a bias correction, whereas the other three models performed reasonably well. The ANN model's performance was highest, with accuracies in the range of 95-98%. At little expense to model accuracy, the number of input nodes to the ANN model could be reduced from 223 to 111, provided the full wavelength range was preserved.