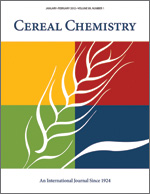
Cereal Chem 72:317-319 |
VIEW ARTICLE
Classification of Hard Red Wheat by Feedforward Backpropagation Neural Networks.
Y. R. Chen, S. R. Delwiche, and W. R. Hruschka. Copyright 1995 by the American Association of Cereal Chemists, Inc.
Because of breeding practices, visual determination of kernel morphology is becoming less dependable for classification of hard red wheat as winter or spring. Because of the price differential between these classes, it is important to develop rapid, accurate, and automatable alternative methods. This study was conducted to determine whether feedforward backpropagation neural networks applied to near-infrared diffuse reflectance spectra of ground kernels could perform the classification. The near-infrared diffuse reflectance spectra (1,100-2,500 nm) of 2,403 ground hard red wheat samples representing the United States crop for 1987-1990 were used with commercially available neural network software. Mathematical pretreatments included log10 (1/reflectance) and second differences of the log data. Networks with and without hidden layers were used with various subsets of the full spectral region as inputs. When developed on samples from the 1987-1989 crop years, the best neural network models yielded 97.0 and 96.8% accuracies for calibration and validation sets, respectively, utilizing the full wavelength range. This performance declined slightly to calibration and validation accuracies of 96.3 and 95.9%, respectively, when the wavelength range of 2,142- 2,472 nm was used. When applied to the 1990 crop year, the prediction accuracies of the full and abbreviated wavelength range models were 95.1 and 95.6%, respectively. These models performed better than a previously reported principal component analysis with Mahalanobis distance classifier. Neural networks, combined with second difference pretreatment, should be a very useful component of a near- infrared based classification system.